In an era where artificial intelligence (AI) is heralded as a transformative tool, its application in career planning and professional development faces significant challenges. This article critically explores the limitations of AI-driven tools in addressing the needs of PhD graduates and international job seekers, highlighting biases, cultural insensitivity, and an overemphasis on automation. It emphasizes the necessity of integrating human-centric approaches with AI to create ethical, inclusive, and effective career acceleration programs. The discussion underscores the importance of tailored solutions, such as Technology-Driven Career Acceleration, continuing education and acceleration programs, to meet the evolving demands of a global and diverse workforce.
Dr. Javad Zarbakhsh, Cademix Institute of Technology, Austria
Table of Contents
Introduction
Artificial intelligence (AI) has undeniably become a transformative force in various domains, from healthcare to education, reshaping how problems are approached and solved. Within the field of career planning and professional development, AI-powered tools are often presented as revolutionary solutions, offering services ranging from personalized job recommendations to automated skill assessments. For PhD graduates and international job seekers, who frequently face daunting challenges in translating their expertise into industry-ready profiles, these tools appear to hold great promise. They aim to bridge the gap between the specialized academic training these individuals possess and the practical demands of global industries.
However, the reality is more complex. AI, while powerful, is not a cure-all. The nuanced and highly individualized nature of career transitions reveals the limitations of even the most advanced AI tools. These shortcomings are particularly pronounced for users navigating diverse cultural, geographic, and organizational landscapes. In this context, the need for human-centric approaches that complement technology becomes evident. This article critically examines the transformative potential of AI in career planning while highlighting the critical gaps and barriers that remain, particularly for PhD graduates and international job seekers.
AI in Career Planning: A Transformative Potential
The integration of AI into career planning has introduced unprecedented capabilities. Tools leveraging machine learning algorithms analyze vast datasets to generate tailored career paths, identify skill gaps, and suggest training programs or job opportunities. Platforms such as LinkedIn, which employs AI-driven job recommendation systems, have gained significant traction. Features like personalized resume builders and automated skill assessments promise to make the daunting process of career planning more accessible and efficient.
For PhD graduates transitioning into industry roles, AI tools can serve as initial guides, particularly in identifying industry-aligned roles for highly specialized academic skills. Similarly, international job seekers may benefit from AI tools designed to parse regional job markets and provide insight into trending skills or occupations. These tools are particularly valuable in fast-paced, data-driven industries, where staying informed about evolving demands is essential.
Yet, these benefits are not evenly distributed. AI tools often fail to consider the complex realities of individuals navigating unique career pathways. For instance, while the algorithms may excel in processing data and generating options, they often lack the cultural sensitivity or contextual awareness required to address the needs of international users or those in niche academic fields. Additionally, career planning involves more than data matching; it encompasses psychological preparedness, confidence building, and navigating interpersonal relationships—all aspects that AI alone cannot effectively address.
The Limitations of AI in Career Tools
Lack of Contextual Sensitivity
AI’s inability to fully grasp the contextual nuances of individual users is among its most significant limitations. For international PhD graduates seeking roles in foreign markets, understanding regional job trends, professional norms, and workplace expectations is crucial. However, most AI tools rely on datasets that are primarily reflective of Western markets and practices, limiting their ability to cater to diverse users. For example, a PhD graduate from Asia or Africa applying for positions in Europe may receive recommendations that overlook critical barriers such as visa restrictions, language requirements, or cultural differences in workplace interactions.
This lack of granularity results in generic advice that fails to resonate with users’ specific needs. Instead of empowering users, it can lead to frustration and even disengagement, as the recommendations appear disconnected from their realities. For international job seekers, these shortcomings may further marginalize their efforts to integrate into host job markets effectively.
Biases in AI Algorithms
Bias in AI tools is an ongoing concern, particularly in career planning, where the consequences of biased recommendations can be profound. AI systems often rely on historical employment data, which may inadvertently encode systemic inequalities. For instance, algorithms trained on Western-centric datasets may undervalue qualifications from non-Western institutions or default to recommending roles that conform to existing stereotypes about certain demographic groups.
For international job seekers, these biases create additional barriers. A highly qualified professional from a developing country may be funneled into roles that undervalue their expertise, while women in STEM fields may face algorithmic biases that skew recommendations toward traditionally “female-dominated” professions. Such biases not only perpetuate inequities but also damage trust in AI systems, deterring individuals from relying on them for critical career decisions.
Overemphasis on Automation
While automation is a hallmark of AI, its dominance can overshadow the need for personalization. Career planning is an inherently personal process, shaped by an individual’s aspirations, values, and unique circumstances. For PhD graduates, this often includes the challenge of translating their niche academic expertise into broadly applicable industry skills. Automation, while efficient, struggles to capture these nuances.
For instance, a PhD graduate in theoretical physics seeking a role in finance may require tailored advice on framing their skills in data analysis and quantitative modeling for non-academic contexts. AI tools, constrained by their reliance on predefined patterns, often fall short of providing the depth and specificity needed for such transitions. This overreliance on automation can leave users feeling unsupported, especially when navigating complex or unconventional career paths.
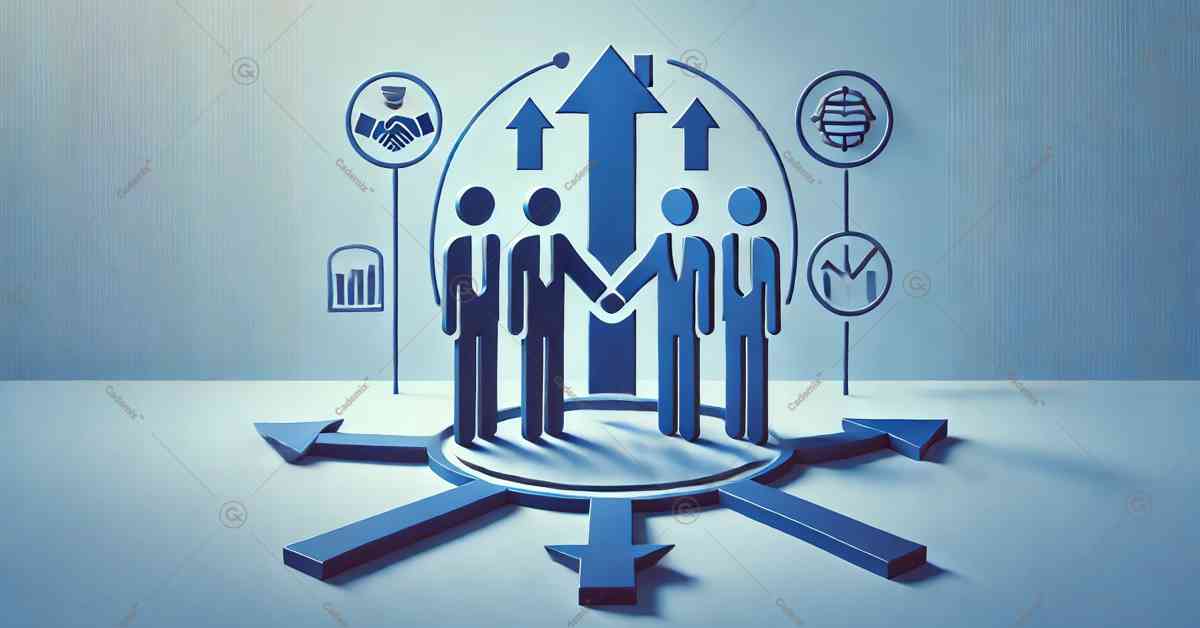
Integrating AI with Human-Centric Approaches
The integration of AI with human-centric approaches offers a pathway to overcoming many of the challenges faced by PhD graduates and international job seekers. While AI tools bring unmatched efficiency and scalability, they often lack the nuanced understanding required to address individual needs and complex career transitions. By combining the capabilities of AI with the insights and empathy of human advisors, a more holistic and effective career planning framework can be developed.
The Role of Human Advisors
AI tools excel at analyzing vast amounts of data, identifying trends, and providing general recommendations. However, their limitations become evident in scenarios requiring empathy, intuition, and contextual judgment. Human advisors—whether mentors, career coaches, or industry experts—play a critical role in filling these gaps. They bring a level of emotional intelligence that AI cannot replicate, offering personalized advice, encouragement, and strategies for overcoming unique challenges.
For example, mentors can help job seekers frame their narratives, aligning their academic achievements with industry expectations in ways that resonate with hiring managers. A PhD graduate specializing in theoretical physics might struggle to convey how their analytical skills translate into roles in data science or finance. A mentor can help craft this translation, contextualizing it for specific industries and roles. Additionally, mentors provide guidance on professional etiquette, cultural adaptation, and navigating workplace politics—areas where AI tools often fall short.
Another crucial aspect of human involvement is the ability to provide constructive feedback and foster resilience. Career transitions, especially for international job seekers, are fraught with rejections and setbacks. Human advisors can offer the moral support and tailored strategies needed to keep individuals motivated and on track, ensuring they do not abandon their career aspirations due to temporary challenges.
Tailoring AI for Diverse Workforce Needs
For AI to serve as a truly effective career development tool, it must be tailored to meet the needs of a diverse and global workforce. Current AI systems often rely on datasets that are limited in scope, reflecting biases inherent in their design. For instance, an AI tool trained predominantly on datasets from Western job markets may fail to account for the unique challenges faced by job seekers from developing countries or those transitioning into roles in non-Western regions.
To address these shortcomings, AI developers must prioritize inclusivity and representation in their algorithms. This involves training AI models on datasets that encompass a wide range of cultural, geographic, and industry-specific contexts. Such diversity ensures that AI recommendations are relevant and equitable for job seekers across different backgrounds. For example, an AI tool designed to assist international job seekers must consider factors like language proficiency, visa requirements, and cultural differences in professional communication styles.
In addition to improving datasets, AI systems should incorporate mechanisms to actively mitigate biases. This requires collaboration with experts in organizational psychology, human resource management, and ethics. By designing transparent AI frameworks that prioritize fairness and accountability, developers can build tools that inspire trust among users. For example, an AI-powered resume screening tool should not only identify qualifications but also flag potential biases in the hiring process, offering recommendations to create a more inclusive recruitment strategy.
Enhancing Collaboration Between AI and Human Advisors
The synergy between AI tools and human advisors can be further enhanced through structured collaboration. AI systems can serve as an initial filter, identifying key trends, skills gaps, and job opportunities. Human advisors can then step in to interpret these insights, providing the personal touch needed to refine strategies and address individual concerns.
For instance, AI can analyze a job seeker’s LinkedIn profile, identifying areas for improvement or suggesting connections to industry professionals. A career coach can then work with the individual to craft personalized messages, prepare for networking events, or develop a tailored job application strategy. This collaborative approach ensures that job seekers benefit from both the efficiency of AI and the nuanced guidance of human mentors.
Moreover, structured collaboration can extend to institutions and organizations. Universities, for example, can integrate AI tools into their career services while training staff to complement these tools with human-centric support. Similarly, industries can use AI to streamline recruitment processes, while maintaining human oversight to ensure fairness and inclusivity.
Building Resilience Through Hybrid Models
One of the most significant advantages of integrating AI with human-centric approaches is the ability to build resilience among job seekers. Career transitions often involve uncertainty, rejection, and self-doubt. While AI tools provide data-driven insights, they lack the capacity to address the emotional toll of these experiences.
Human advisors, on the other hand, can help individuals develop the resilience needed to navigate these challenges. By fostering a growth mindset and encouraging continuous learning, mentors can empower job seekers to adapt to changing circumstances. This is particularly important for international graduates, who may face additional hurdles such as adapting to new cultural norms or dealing with discrimination in the workplace.
The hybrid model also promotes lifelong learning, encouraging individuals to use AI tools for skill development while seeking mentorship for career planning and personal growth. This combination ensures that job seekers are not only prepared for their immediate transitions but are also equipped to thrive in an evolving job market.
By integrating AI and human-centric approaches, career development frameworks can address both the practical and emotional dimensions of career transitions. This hybrid model represents the future of career planning, combining technological innovation with the human touch needed to navigate complex and diverse workforce needs.
Ethical and Inclusive Frameworks for AI Development
Building Trust in AI Tools
Trust in AI tools is not easily earned, especially in fields as personal and impactful as career planning. Users, particularly PhD graduates and international job seekers, often approach these tools with skepticism, questioning their reliability and fairness. This mistrust is exacerbated when ethical principles are overemphasized without tangible evidence of fairness, inclusivity, or transparency. While ethical guidelines are necessary, an overemphasis on them—especially when presented in abstract terms—can inadvertently raise resistance. Some users may perceive such principles as superficial marketing tactics rather than concrete commitments to fairness, leading to doubts about the tool’s real-world applicability and integrity.
Moreover, the ethical conversation must avoid appearing one-sided or overly prescriptive. When ethical considerations dominate discussions without balancing practical usability, they risk alienating users who may see these tools as overly constrained or disconnected from their immediate needs. For AI developers, the challenge lies in demonstrating that ethical principles are embedded in the tool’s design and functionality, not just in rhetoric. This can be achieved by providing users with clear, actionable information about how the AI operates—offering transparency in how recommendations are generated, what data is used, and how potential biases are mitigated. By empowering users with this knowledge, trust can be built incrementally, fostering wider adoption.
The Challenge of Inclusivity in AI Development
AI tools are often designed and trained using datasets derived predominantly from Western contexts, reflecting the values, norms, and economic realities of those societies. While these tools might excel in addressing the needs of users in these regions, their applicability to job seekers in developing countries, Asia, Africa, and other traditional societies is often limited. This lack of inclusivity poses a significant barrier to their adoption in global markets.
For instance, career advice tailored to a U.S.-based job market may emphasize individual achievements and assertiveness—qualities that might align poorly with cultural norms in more collectivist societies, where teamwork and community values are prioritized. Similarly, AI systems might suggest career paths or networking strategies that overlook significant structural differences, such as limited access to industry-specific mentors or professional networks in less industrialized regions.
The reliance on Western-centric data also raises concerns about the exclusion of non-Western qualifications, experiences, and career trajectories. For example, an AI tool might undervalue educational credentials or professional experiences from developing countries simply because they are underrepresented in the training data. This perpetuates systemic inequalities and creates additional hurdles for international job seekers, who may already face biases during recruitment processes.
Overcoming Cultural and Regional Biases
To develop truly inclusive AI tools, developers must expand their datasets to incorporate a broader range of cultural, geographic, and industry-specific contexts. This includes integrating data from underrepresented regions and actively collaborating with local organizations to ensure relevance. For example, AI tools could partner with universities and industries in developing countries to gather data on regional labor markets, qualifications, and professional norms. This approach ensures that AI recommendations are not only globally applicable but also culturally sensitive.
Furthermore, inclusivity must extend beyond data representation to the very design of the tools. Algorithms should be designed to recognize and adapt to cultural nuances, offering recommendations that are tailored to the user’s local context. For instance, a tool could provide job seekers in traditional societies with advice that aligns with local communication styles, workplace hierarchies, and professional expectations. This adaptability requires collaboration between AI developers and experts in organizational psychology, human resource management, and cultural studies.
Addressing Resistance Through Practical Solutions
While inclusivity and ethics are essential, their overemphasis can create unintended resistance among users who perceive these values as obstacles to innovation or pragmatism. For some, the emphasis on fairness and inclusivity may raise concerns about compromising the tool’s efficiency or effectiveness. To counteract this resistance, developers must demonstrate that ethical and inclusive practices enhance the user experience rather than hinder it. For example, showcasing case studies where inclusive AI systems have successfully supported diverse job seekers can help bridge this gap.
In addition, offering customization options can address concerns about the tool’s adaptability to local contexts. Users should be able to personalize settings, choose preferred metrics, and provide feedback on the tool’s performance. These features not only improve usability but also empower users, giving them a sense of ownership and control over their career planning process.
Proactive Measures for Equitable AI Tools
Developers must also take proactive steps to address systemic inequalities in the labor market. This involves designing AI tools that actively support underrepresented groups, such as women in STEM, individuals from economically disadvantaged regions, or professionals transitioning between vastly different career paths. Features like targeted training modules, region-specific career advice, and mentorship programs can complement AI capabilities, creating a more equitable ecosystem.
For instance, an AI tool designed for international job seekers could include modules on navigating visa requirements, adapting to workplace cultures, and identifying industries with high demand for their skills. Paired with human mentorship, these features can help bridge the gap between systemic challenges and individual career aspirations, ensuring that users receive comprehensive and practical support.
A Call for Transparent and Inclusive AI Frameworks
To truly achieve inclusivity, AI frameworks must prioritize transparency and accountability. Developers should actively engage with diverse stakeholders, including international job seekers, regional experts, and policymakers, during the design process. This collaborative approach ensures that ethical principles are not just theoretical constructs but practical elements embedded in the tool’s functionality.
Transparency also involves making the limitations of AI tools explicit. Users should be informed about potential biases, gaps in the data, and areas where human input is essential. By managing expectations and fostering an open dialogue, developers can build trust and encourage constructive feedback, creating a continuous improvement loop.
In conclusion, while ethics and inclusivity are cornerstones of responsible AI development, their implementation must go beyond rhetoric. By addressing the unique challenges faced by diverse and global users, developers can create AI tools that are not only ethical but also practical, relevant, and transformative.
Challenges for PhD Graduates and International Job Seekers
PhD graduates transitioning to industry roles face a complex web of challenges, many of which cannot be fully addressed by AI-driven tools alone. While these tools can assist with tasks such as resume optimization, job matching, and skill assessment, they often fall short in navigating the nuanced, human-centric aspects of career transitions. For instance, PhD holders often struggle with cultural mismatches between the academic environment—where long-term, hypothesis-driven research dominates—and corporate settings that prioritize immediate results, collaboration, and applied skills. This disconnect is particularly pronounced for those whose academic work was deeply theoretical or specialized, as they may find it difficult to articulate the broader applicability of their expertise in industry terms.
For international job seekers and graduates, the challenges are magnified by their need to adapt to foreign workplace cultures and norms. Language barriers, unfamiliar social customs, and differing expectations in workplace hierarchies can make integration into a new workforce daunting. These issues are compounded by a lack of understanding of local job market dynamics, including industry-specific demands, recruitment practices, and networking strategies that may differ significantly from those in their home countries. AI tools, while helpful for general guidance, often fail to account for these localized nuances, leaving international job seekers at a disadvantage.
A hybrid approach is critical in addressing these multifaceted challenges. While AI can provide scalable solutions for skill development, job matching, and professional branding, regular meetings with a mentor are essential for personalized guidance. Ideally, such a mentor should have an international background or experience working with diverse groups, enabling them to offer insights into the cultural and professional nuances of the host country. These mentors can help bridge the gap by providing tailored advice on adapting to local workplace norms, overcoming cultural barriers, and identifying opportunities for leveraging unique international perspectives.
Moreover, mentors can offer emotional and professional support, which is often overlooked in purely technological solutions. The transition from academia to industry, particularly in a foreign country, can be an isolating and stressful experience. Regular interactions with a mentor can provide a sense of continuity, encouragement, and a safe space for discussing uncertainties. This personalized guidance, paired with high-tech tools that focus on practical applications like skill matching and career tracking, creates a synergistic framework. Such an approach not only prepares job seekers for the immediate challenges of integration but also empowers them to navigate their careers with confidence and adaptability in the long term.
Recommendations for the Future
The future of AI-driven career tools lies in their ability to complement, rather than replace, human expertise. To achieve this, stakeholders across academia, industry, and technology development must collaborate to create integrated solutions. Universities and research institutions can play a pivotal role by incorporating career planning modules into their curricula, emphasizing the use of AI alongside traditional mentorship. Industry leaders, meanwhile, can contribute by providing real-world datasets and insights to enhance the relevance of AI tools.
Programs like acceleration initiatives and continuing education workshops offered by organizations such as the Cademix Institute of Technology serve as exemplary models. These programs bridge the gap between academic training and industry demands, equipping participants with the skills and resilience needed to thrive in a rapidly evolving job market.
Toward a Balanced Approach
While AI represents a significant advancement in career planning, it is not a standalone solution. Its limitations underscore the need for a balanced approach that integrates technology with human-centric strategies. By addressing the biases, contextual gaps, and over-reliance on automation that currently hinder AI tools, we can create a system that empowers job seekers—particularly PhD graduates and international professionals—to navigate the complexities of the modern workforce effectively.
References and Further Reading
- Binns, R. (2018). Fairness in Machine Learning: Lessons from Political Philosophy. Proceedings of the 2018 Conference on Fairness, Accountability, and Transparency. Link
- Veletsianos, G. (2016). Social Media in Academia: Networked Scholars. Routledge. Link
- Eubanks, V. (2018). Automating Inequality: How High-Tech Tools Profile, Police, and Punish the Poor. St. Martin’s Press. Link
- Sugimoto, C. R., Work, S., Larivière, V., & Haustein, S. (2017). Scholarly Use of Social Media and Altmetrics: A Review of the Literature. Journal of the Association for Information Science and Technology, 68(9), 2037–2062. DOI: 10.1002/asi.23833
- Binns, V. H., & Jensen, S. H. (2020). AI Bias in Recruitment Tools: Systemic Challenges and Opportunities for Equity. AI and Society. DOI: 10.1007/s00146-020-00999-3
- Haustein, S., Peters, I., Sugimoto, C. R., Thelwall, M., & Larivière, V. (2014). Tweets as Impact Indicators: Examining the Implications of Social Media for Scholarly Communication. Journal of the Association for Information Science and Technology, 65(4), 656–669. DOI: 10.1002/asi.23101
- Bik, H. M., & Goldstein, M. C. (2013). An Introduction to Social Media for Scientists. PLoS Biology, 11(4), e1001535. DOI: 10.1371/journal.pbio.1001535
- McClain, C. R., & Neeley, L. (2015). A Critical Evaluation of Science Outreach via Social Media: Its Role and Impact on Scientists. F1000Research, 3, 300. DOI: 10.12688/f1000research.5918.1