Artificial intelligence has transitioned from a back-end computational tool to a pervasive force shaping how societies make decisions, consume information, and form opinions. Algorithms that once merely sorted data or recommended music now influence hiring outcomes, political discourse, medical diagnoses, and patterns of consumer spending. This shift toward AI-driven influence holds remarkable promise, offering efficiency, personalization, and consistency in decision-making processes. Yet it also raises a host of moral dilemmas. The capacity of AI to guide human choices not only challenges core ethical principles such as autonomy, transparency, and fairness but also raises urgent questions about accountability and societal values. While many hail AI as the next frontier of progress, there is growing recognition that uncritical reliance on automated judgments can erode trust, entrench biases, and reduce individuals to subjects of algorithmic persuasion.
Keyphrases: AI Ethics and Influence, Automated Decision-Making, Responsible AI Development
Table of Contents
Abstract
The expanding role of artificial intelligence in shaping decisions—whether commercial, political, or personal—has significant ethical ramifications. AI systems do more than offer suggestions; they can sway public opinion, limit user choices, and redefine norms of responsibility and agency. Autonomy is imperiled when AI-driven recommendations become so persuasive that individuals effectively surrender independent judgment. Transparency is likewise at risk when machine-learning models operate as black boxes, leaving users to question the legitimacy of outcomes they cannot fully understand. This article dissects the ethical quandaries posed by AI’s increasing influence, examining how these technologies can both serve and undermine human values. We explore the regulatory frameworks emerging around the world, analyze real-world cases in which AI’s power has already tested ethical boundaries, and propose a set of guiding principles for developers, policymakers, and end-users who seek to ensure that automated decision-making remains consistent with democratic ideals and moral imperatives.
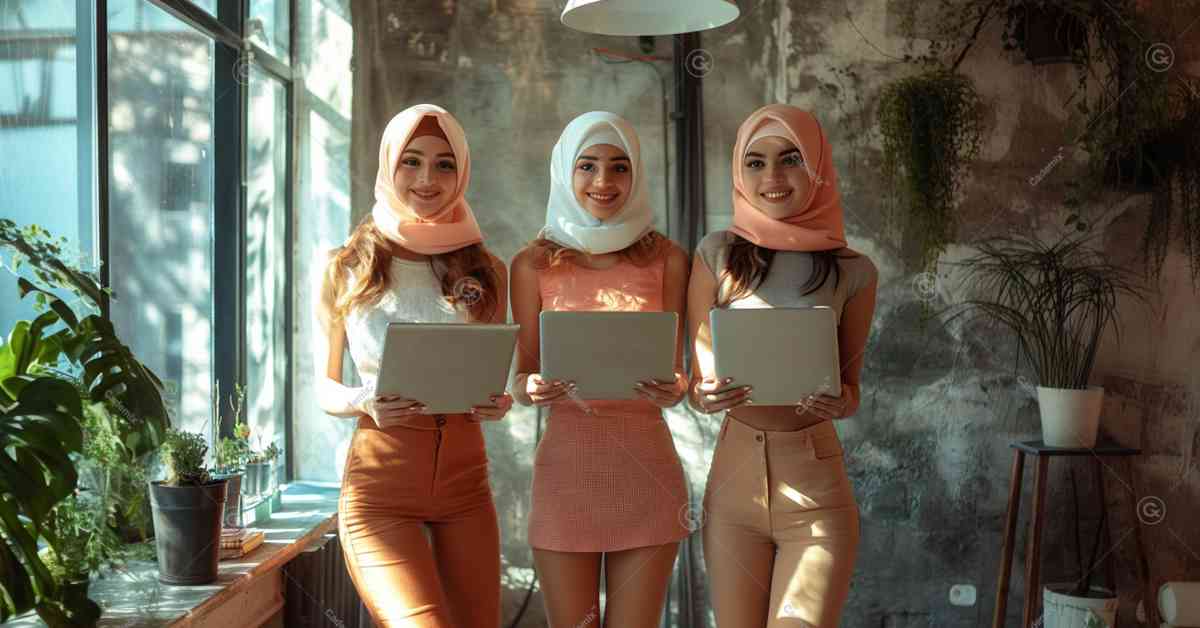
Introduction
Recent years have seen a surge in AI adoption across various domains, from software systems that rank job applicants based on video interviews to chatbots that guide patients through mental health screenings. The impetus behind this shift often centers on efficiency: AI can rapidly sift through troves of data, detect patterns invisible to human analysts, and deliver results in fractions of a second. As a result, businesses and governments alike view these systems as powerful enablers of growth, cost-saving measures, and enhanced service delivery. However, the conversation about AI’s broader implications is no longer confined to performance metrics and cost-benefit analyses.
One focal concern involves the subtle yet profound ways in which AI can reshape human agency. When an algorithm uses user data to predict preferences and behaviors, and then tailors outputs to produce specific responses, it ventures beyond mere assistance. It begins to act as a persuader, nudging individuals in directions they might not have consciously chosen. This is particularly visible in social media, where content feeds are algorithmically personalized to prolong engagement. Users may not realize that the stories, articles, or videos appearing on their timeline are curated by machine-learning models designed to exploit their cognitive and emotional responses. The ethics of nudging by non-human agents become even more complicated when the “end goal” is profit or political influence, rather than a user’s stated best interest.
In tandem with these manipulative potentials, AI systems pose challenges around accountability. Traditional frameworks for assigning blame or liability are premised on the idea that a human or organization can be identified as the primary actor in a harmful incident. But what happens when an AI model recommended an action or took an automated step that precipitated damage? Software developers might claim they merely wrote the code; data scientists might say they only trained the model; corporate executives might argue that the final decisions lay with the human operators overseeing the system. Legal scholars and ethicists debate whether it makes sense to speak of an algorithm “deciding” in a moral sense, and if so, whether the algorithm itself—lacking consciousness and moral judgment—can be held responsible.
Another ethical question revolves around transparency. Machine-learning models, particularly neural networks, often function as opaque systems that are difficult even for their creators to interpret. This opacity creates dilemmas for end-users who might want to challenge or understand an AI-driven outcome. A loan applicant denied credit due to an automated scoring process may justifiably ask why. If the system cannot provide an understandable rationale, trust in technology erodes. In crucial applications such as healthcare diagnostics or criminal sentencing recommendations, a black-box approach can undermine essential democratic principles, including the right to due process and the idea that public institutions should operate with a degree of openness.
These tensions converge around a central theme: AI’s capacity to influence has outpaced the evolution of our ethical and legal frameworks. While “human in the loop” requirements have become a popular safeguard, simply having an individual rubber-stamp an AI recommendation may not suffice, especially if the magnitude of data or complexity of the model defies human comprehension. In such scenarios, the human overseer can become a figurehead, unable to truly parse or challenge the system’s logic. Addressing these concerns demands a deeper exploration of how to craft AI that respects user autonomy, ensures accountability, and aligns with societal norms. This article contends that the path forward must integrate technical solutions—like explainable AI and rigorous audits—with robust policy measures and a culturally entrenched ethics of technology use.
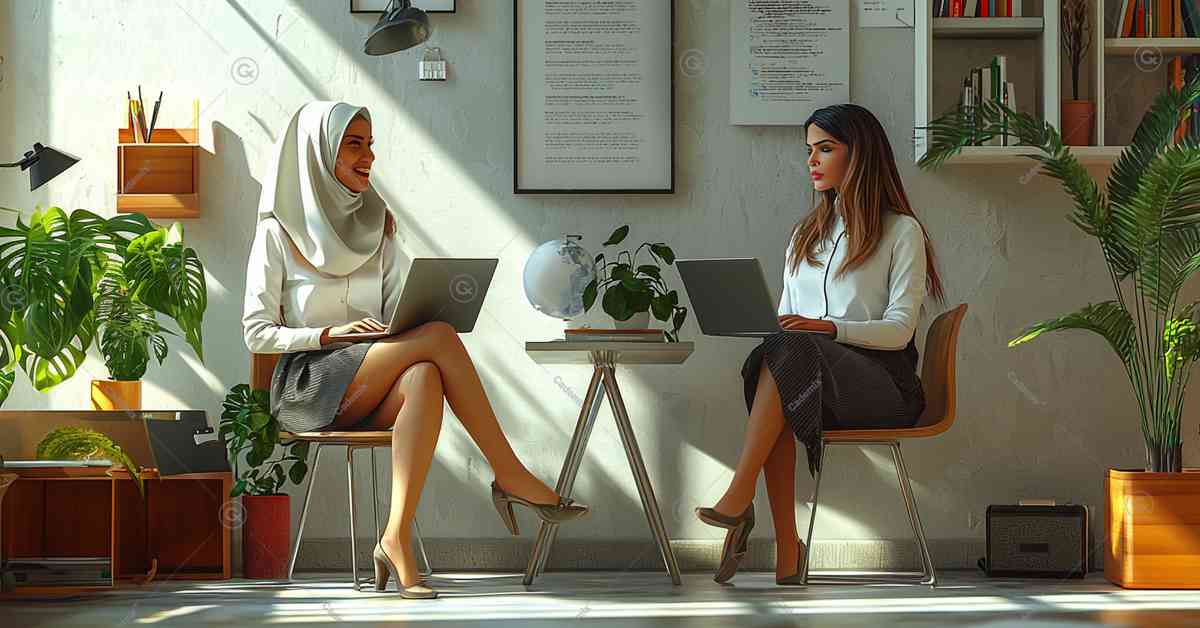
The Expanding Role of AI in Decision-Making
AI-driven technology has rapidly moved from specialized laboratory research to everyday consumer and enterprise applications. In the commercial arena, algorithms shape user experiences by deciding which products to recommend, which advertisements to display, or which customers to target with promotional offers. On content platforms, “engagement optimization” has become the linchpin of success, with AI sorting infinite streams of images, videos, and text into personalized feeds. The infiltration of AI goes beyond marketing or entertainment. Hospitals rely on predictive analytics to estimate patient risks, while banks use advanced models to flag suspicious transactions or determine loan eligibility. Political campaigns deploy data-driven persuasion, micro-targeting ads to voters with unprecedented precision.
This ubiquity of AI-based tools promises improved accuracy and personalization. Home security systems can differentiate residents from intruders more swiftly, supply chains can adjust in real time based on predictive shipping patterns, and language translation software can bridge communications across cultures instantly. Yet at the core of these transformations lies a subtle shift in the locus of control. While humans nominally remain “in charge,” the scale and speed at which AI processes data mean that individuals often delegate significant portions of decision-making to algorithms. This delegation can be benign—for example, letting an app plan a driving route—until it encounters ethically charged territory such as a social media platform inadvertently promoting harmful misinformation.
Crucial, too, is the competitive pressure fueling rapid deployment. Businesses that fail to harness AI risk being outmaneuvered by rivals with more data-driven insights. Public sector institutions also face pressure to modernize, adopting AI tools to streamline services. In this race to remain relevant, thorough ethical assessments sometimes fall by the wayside, or become tick-box exercises rather than genuine introspection. The consequences emerge slowly but visibly, from online recommendation systems that intensify political polarization to job application portals that penalize candidates whose backgrounds deviate from historical norms.
One of the more insidious aspects of AI influence is that its footprints can be undetected by most users. Because so many machine-learning models operate under the hood, the impetus or logic behind a particular suggestion or decision is rarely visible. An online shopper might merely note that certain items are suggested, or a social media user might see certain posts featured prominently. Unaware that an AI system orchestrates these experiences, individuals may not question the nature of the influence or understand how it was derived. Compounded billions of times daily, these small manipulations culminate in large-scale shifts in economic, cultural, and political spheres.
In environments where personal data is abundant, these algorithms become exceptionally potent. The more the system knows about a user’s preferences, browsing history, demographic profile, and social circles, the more precisely it can tailor its outputs to produce desired outcomes—be they additional sales, content engagement, or ideological alignment. This dynamic introduces fundamental ethical questions: does an entity with extensive knowledge of an individual’s behavioral triggers owe special duties of care or impose particular forms of consent? Should data-mining techniques that power these recommendation systems require explicit user understanding and approval? As AI weaves itself deeper into the structures of daily life, these concerns about autonomy and awareness grow pressing.
Ethical Dilemmas in AI Influence
The moral landscape surrounding AI influence is complex and multifaceted. One of the central dilemmas concerns autonomy. Individuals pride themselves on their capacity to make reasoned choices. Yet AI-based recommendation engines, social media feeds, and search rankings can guide their options to such an extent that free will becomes blurred. When everything from the news articles one sees to the job openings one learns about is mediated by an opaque system, the user’s agency is subtly circumscribed by algorithmic logic. Ethicists question whether this diminishes personal responsibility and fosters dependency on technology to make choices.
A second tension arises between beneficial persuasion and manipulative influence. Persuasion can serve positive ends, as when an AI system encourages a patient to adopt healthier behaviors or helps a student discover relevant scholarship opportunities. But manipulation occurs when the system capitalizes on psychological vulnerabilities or incomplete information to steer decisions that are not truly in the user’s best interest. The boundary between the two can be elusive, particularly given that AI tailors its interventions so precisely, analyzing emotional states, time of day, or user fatigue to optimize engagement.
Bias remains another critical concern. As outlined in the preceding article on AI bias, prejudiced data sets or flawed design choices can yield discriminatory outcomes. When these biases combine with AI’s capacity to influence, entire demographic groups may face systematic disadvantages. An example is job recruitment algorithms that favor certain racial or gender profiles based on historical patterns, effectively locking out other candidates from key opportunities. If these processes operate behind the scenes, the affected individuals may not even realize that they were subject to biased gatekeeping, compounding the injustice.
Questions about liability also loom large. Although an AI system may produce harmful or ethically dubious results, it remains a product of collaborative design, training, and deployment. Identifying who bears moral or legal responsibility can be difficult. The software vendor might disclaim liability by citing that they provided only a tool; the user might rely on the tool’s recommendations without scrutiny; the data providers might have contributed biased or incomplete sets. This diffusion of accountability undermines traditional frameworks, which rely on pinpointing a responsible party to rectify or prevent harm. For AI to operate ethically, a new model for allocating responsibility may be necessary—one that accommodates the distributed nature of AI development and use.
Finally, transparency and explainability surface as ethical imperatives. If an individual’s future is materially impacted by an AI decision—for instance, if they are denied a mortgage, rejected for a job, or flagged by law enforcement—they arguably deserve a comprehensible explanation. Without it, recourse or appeal becomes nearly impossible. Yet many sophisticated AI systems, especially deep learning architectures, cannot readily articulate how they arrived at a given conclusion. This opacity threatens fundamental rights and can corrode trust in institutions that outsource major judgments to inscrutable algorithms.
Regulatory Approaches to AI Ethics
As AI’s capacity for influence expands, governments, international bodies, and private-sector stakeholders have begun proposing or implementing frameworks to ensure responsible use. These efforts range from broad ethical principles to legally binding regulations. In the European Union, the proposed AI Act aims to classify AI systems by risk level, imposing stricter requirements on high-risk applications such as biometric surveillance or systems used in critical infrastructure. Similar guidelines exist in other regions, though the degree of enforcement varies widely.
The United States, while lacking comprehensive federal AI legislation, has witnessed calls for policy reform. The White House unveiled a Blueprint for an AI Bill of Rights, advocating for principles such as safe and effective systems, data privacy, and protection from abusive data practices. Meanwhile, state-level measures address specific concerns, like prohibiting the use of facial recognition by law enforcement. Major technology companies have also launched their own ethical codes of conduct, an acknowledgment that self-regulation might be necessary to stave off more punitive government oversight.
China presents a contrasting regulatory model, as the government places strong emphasis on national security and social stability. AI governance there can be more stringent and centralized, with heavy scrutiny over technologies that track citizens’ movements or shape public opinion. The ethical dimension merges with the political, raising unique concerns over privacy, censorship, and state-driven manipulations.
Non-governmental organizations and research consortia have stepped into the vacuum to offer standard-setting guidelines. The Institute of Electrical and Electronics Engineers (IEEE) has championed frameworks for ethical AI design, focusing on accountability, transparency, and harm mitigation. The Partnership on AI, an international consortium including technology giants and civil society groups, publishes best practices and fosters dialogue between diverse stakeholders. Yet, a consistent challenge remains: how to translate aspirational principles into enforced regulations and daily operational changes.
One emerging idea is to require “algorithmic impact assessments,” similar to environmental impact statements. These assessments would mandate that organizations deploying AI systems, especially in sensitive areas, evaluate potential risks to civil liberties, fairness, and user autonomy. The assessment process would also encourage public consultation or expert review. Another approach calls for robust auditing procedures, potentially administered by independent external bodies. In such a model, algorithms that shape public discourse or critical life decisions would undergo periodic evaluations for bias, manipulative tendencies, or hidden conflicts of interest. While these proposals carry promise, they also raise questions about feasibility, cost, and the boundary between corporate confidentiality and public oversight.
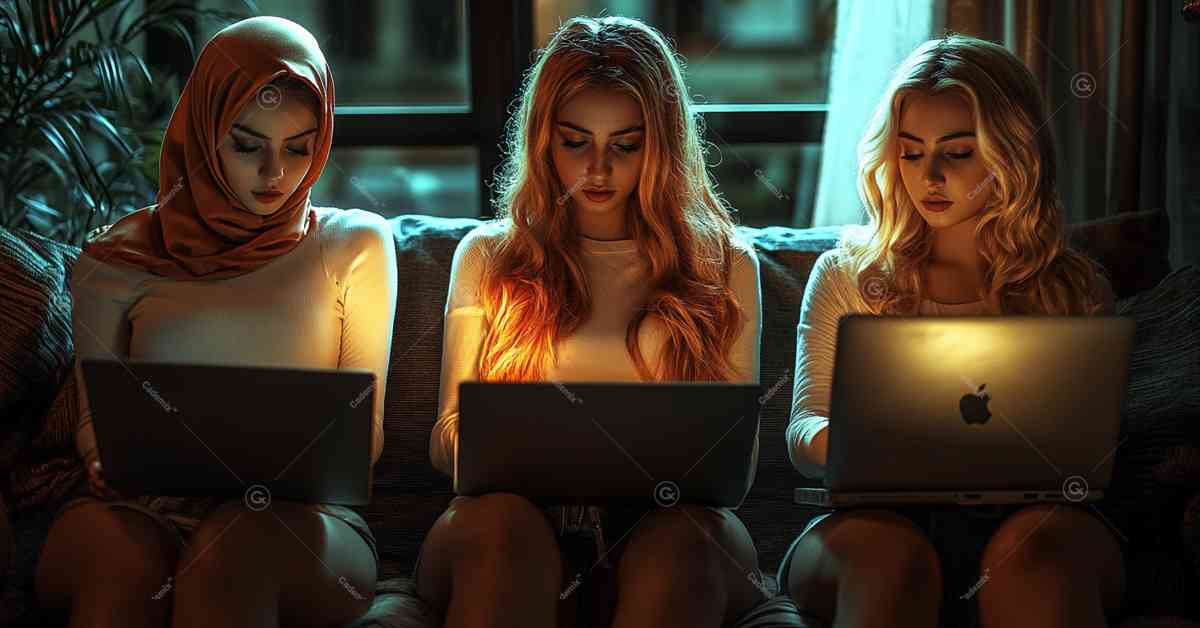
Strategies for Ethical AI Development
Ensuring that AI influence aligns with human values and fosters trust requires a blend of technical innovation, organizational culture change, and continuous vigilance. One foundational concept is “ethical AI by design.” Rather than retrofitting moral safeguards after a product has been built and launched, developers and stakeholders incorporate ethical considerations from the earliest stages of ideation. This approach compels data scientists to carefully select training sets, engineers to embed transparency features, and project managers to define success metrics that include social impact.
In parallel, bias audits and iterative evaluations can identify harmful patterns before they become entrenched. Teams can analyze how an AI system performs across demographics, verifying whether certain outcomes cluster disproportionately among minority populations or vulnerable groups. If discovered, these disparities prompt re-training with more representative data or adjustments to the model’s architecture. By publicizing the audit results and remedial measures, organizations can signal accountability and bolster user confidence.
Human oversight remains critical in many high-stakes applications. Whether in loan approvals, medical diagnoses, or law enforcement, the final say might rest with a trained professional who can override an AI recommendation. This arrangement, however, only works if the human overseer has both the expertise and the authority to meaningfully challenge the algorithm. Requiring a human signature means little if that person is encouraged, by time constraints or organizational culture, to default to the AI’s judgment. For real accountability, institutions must empower these overseers to question or adapt the algorithm’s output when it seems misaligned with the facts at hand.
Methods that enhance AI interpretability can also deter manipulative or unethical uses. Explainable AI research has made strides in producing visualizations or simplified models that approximate how complex neural networks arrive at decisions. These techniques might highlight which inputs the model weighed most heavily, or provide hypothetical scenarios (“counterfactuals”) that show how changing certain variables would alter the outcome. Although such explanations do not always capture the full complexity of machine learning processes, they can serve as an important communication bridge, allowing non-technical stakeholders to gauge whether the system’s logic is sensible and fair.
Developers and policymakers likewise recognize the importance of user empowerment. Providing individuals with control over their data, letting them opt out of certain AI-driven recommendations, or offering the right to contest algorithmic decisions fosters a sense of agency. In certain industries, a “human in the loop” approach can be complemented by a “user in the loop” model, where end-users have insight into how and why an AI made a particular suggestion. This does not merely quell fears; it can also spur innovative uses of technology, as informed users harness AI capabilities while remaining cautious about potential pitfalls.
Finally, open AI governance models that invite cross-disciplinary participation can mitigate ethical lapses. Sociologists, psychologists, ethicists, and community representatives can all provide perspectives on how AI systems might be interpreted or misused outside the tech bubble. Collaborative design fosters inclusivity, ensuring that concerns about language barriers, cultural norms, or historical injustices are addressed in the engineering process. Such engagement can be formalized through advisory boards or public consultations, making it harder for developers to claim ignorance of an AI system’s real-world ramifications.
The Future of AI Influence
The trajectory of AI influence will likely reflect further advances in deep learning, natural language processing, and sensor fusion that enable systems to integrate physical and digital data seamlessly. Automated agents could become so adept at perceiving user needs and context that they effectively become co-decision-makers, forecasting what we want before we articulate it. In healthcare, for example, predictive analytics might guide every aspect of diagnosis and treatment, delivering personalized care plans. In the corporate realm, AI might orchestrate entire business strategies, from supply chain logistics to marketing campaigns, adapting in real time to market fluctuations.
Such scenarios can be thrilling, as they promise unprecedented convenience and problem-solving capacity. But they also foreground ethical queries. As AI gains the capacity to engage in persuasive interactions that mimic human empathy or emotional intelligence, where do we draw the line between supportive guidance and manipulative conduct? Will chatbots become “digital confidants,” leading vulnerable users down paths that serve corporate interests rather than personal well-being? Society must contend with whether perpetual connectivity and algorithmic oversight risk turning human experience into something algorithmically curated, with diminishing room for spontaneity or dissent.
Regulatory frameworks may grow more robust, particularly as sensational incidents of AI misuse capture public attention. Tools like deepfakes or automated disinformation campaigns highlight how advanced AI can be weaponized to distort truth, sway elections, or harm reputations. Governments may respond by mandating traceable “digital signatures” for AI-generated media, requiring organizations to demonstrate that their content is authentic. Meanwhile, an emphasis on ethics training for engineers and data scientists could become standard in technical education, instilling an ethos of responsibility from the outset.
A shift toward collaborative AI is also plausible. Rather than passively allowing an algorithm to define choices, individuals might engage in iterative dialogues with AI agents, refining their objectives and moral preferences. This approach reframes AI not as a controlling force but as a partner in rational deliberation, where the system’s vast computational resources complement the user’s personal experiences and moral judgments. Achieving this synergy will depend on AI developers prioritizing user interpretability and customizability, ensuring that each person can calibrate how strongly they want an algorithm to shape their decisions.
Public awareness and AI literacy will remain key. If citizens and consumers understand how AI works, what data it uses, and what objectives it pursues, they are more likely to spot manipulative patterns or refuse exploitative services. Educational initiatives, from elementary schools to adult learning platforms, can demystify terms like “algorithmic bias” or “predictive modeling,” equipping individuals with the conceptual tools to assess the trustworthiness of AI systems. In an era when technology evolves more swiftly than legislative processes, an informed public may be the best bulwark against unchecked AI influence.
Conclusion
Artificial intelligence, once a specialized field of computer science, has become a decisive force capable of shaping how societies allocate resources, exchange ideas, and even perceive reality itself. The potent influence wielded by AI is not inherently beneficial or harmful; it is contingent upon the ethical frameworks and design philosophies guiding its development and implementation. As we have seen, the dilemmas are manifold: user autonomy clashes with the potential for manipulation, black-box decision-making challenges transparency, and accountability evaporates when responsibility is diffusely spread across code writers, data providers, and end-users.
Far from recommending a retreat from automation, this article suggests that AI’s future role in decision-making must be governed by safeguards that respect human dignity, equality, and freedom. The task demands a delicate balance. Overregulation may stifle innovation and hamper beneficial applications of AI. Underregulation, however, risks letting clandestine or unscrupulous actors exploit public vulnerabilities, or letting unintended algorithmic biases shape entire policy domains. Achieving equilibrium requires an ecosystem of engagement that includes governments, technology companies, civil society, and everyday citizens.
Responsible AI design emerges as a core strategy for mitigating ethical hazards. By integrating moral considerations from the earliest design stages, performing bias audits, enabling user oversight, and ensuring accountability through transparent practices, developers can produce systems that enhance rather than undermine trust. Organizational and legal structures must then reinforce these best practices, harnessing audits, algorithmic impact assessments, and public disclosure to maintain vigilance. Over time, these measures can cultivate a culture in which AI is perceived as a genuinely assistive partner, facilitating informed choices rather than constraining them.
In essence, the future of AI influence stands at a crossroads. On one path, automation might further entrench power imbalances, fueling skepticism, eroding individual autonomy, and perpetuating societal divides. On the other path, AI could serve as a catalyst for equity, insight, and compassionate governance, augmenting human capacities rather than supplanting them. The direction we take depends on the ethical commitments made today, in the design labs, legislative halls, and public dialogues that define the trajectory of this transformative technology. The choice, and responsibility, ultimately belong to us all.