Estimated Reading Time: 16 minutes A comprehensive post-event evaluation is essential in event management, as it enables continuous improvement, optimizes future event planning, and ensures stakeholder satisfaction by analyzing feedback, metrics, and trends
The Future of Event Planning: Trends to Watch in 2024 and Beyond
Estimated Reading Time: 16 minutes Discover how emerging future of event planning trends like hybrid events, AI integration, and experiential marketing are revolutionizing Event Management in 2024 and beyond.
Comprehensive Guide to Developing Interview Skills: How to Prepare and Succeed in Job Interviews
Estimated Reading Time: 6 minutes Effective interview skills are crucial in the job search process. This comprehensive guide will help you understand how to prepare for interviews, develop strong interview skills, and succeed in your job applications.
Sustainability in Event Planning: How to Go Green
Estimated Reading Time: 16 minutes Sustainability in Event Planning: How to Go Green” explores essential strategies for integrating eco-friendly practices into event management, from selecting sustainable venues to reducing waste and carbon footprints, while balancing budget considerations and embracing the latest event trends.
Chat GPT: Revolutionizing Conversational AI
Estimated Reading Time: 6 minutes Chat GPT, developed by OpenAI, is a groundbreaking advancement in conversational artificial intelligence. As part of the Generative Pre-trained Transformer (GPT) family, Chat GPT excels in generating human-like text, understanding context, and providing accurate responses across a wide range of topics. This article delves into the development, capabilities, applications, and impact of Chat GPT, highlighting its role in transforming how we interact with machines.
AI Image Generation: Advanced Techniques for Optimizing Prompts in ChatGPT and MidJourney
AI Image Generation has become a cornerstone in digital content creation, enabling the production of high-quality visuals with minimal human input. However, the effectiveness of these AI-driven outputs heavily depends on the precision and optimization of prompts, which vary significantly between platforms. This article explores the advanced techniques necessary for optimizing prompts in both ChatGPT and MidJourney, two leading tools in AI-driven image creation. The challenge lies in the distinct requirements of these platforms—where ChatGPT excels in generating conceptual descriptions, MidJourney specializes in translating these into detailed visual content. We address the problem of inconsistent and suboptimal image outputs by examining the intricacies of prompt engineering tailored to each tool, ultimately providing a comprehensive solution for achieving superior AI image generation results.
Abraham Ahmed, Cademix Institute of Technology
Table of Contents
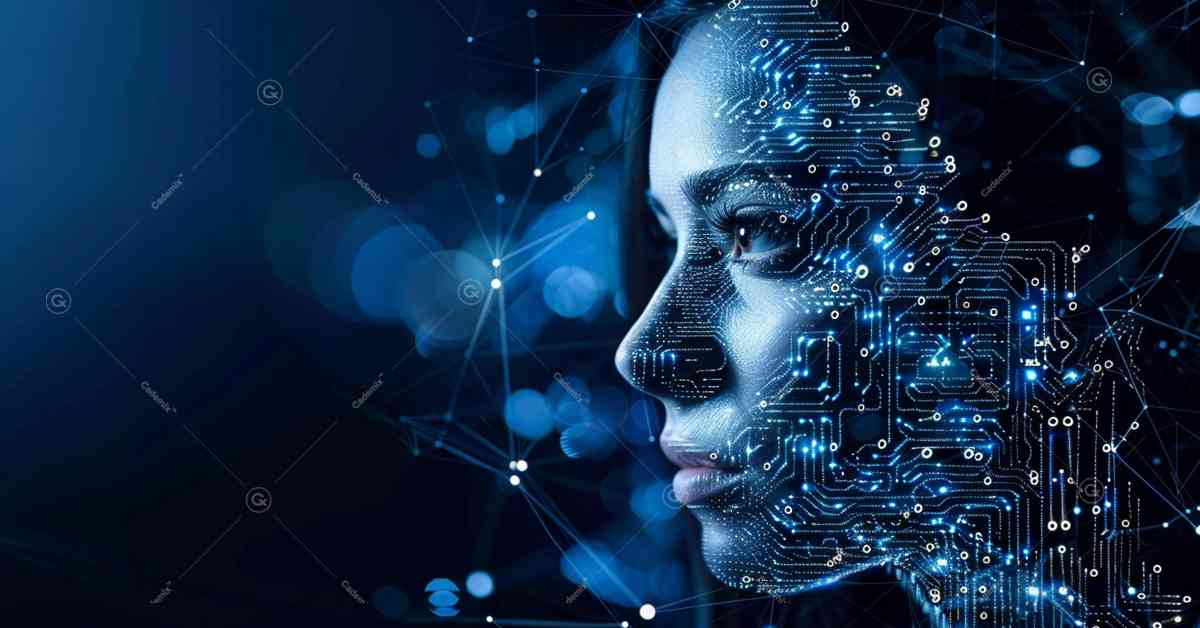
Introduction
AI image generation has emerged as a transformative technology in the digital content creation landscape, enabling creators to produce high-quality visuals with unprecedented ease and speed. This innovation is particularly significant as industries ranging from marketing to entertainment increasingly rely on visually compelling content to engage audiences. However, the process of generating these images is not without its challenges. Central to the effectiveness of AI-driven image creation is the precision with which prompts are crafted and optimized. While artificial intelligence tools like ChatGPT and MidJourney offer incredible potential, they require a deep understanding of their unique capabilities and limitations to maximize their output quality.
At the core of AI image generation is the interplay between textual input and visual output. ChatGPT, a powerful language model developed by OpenAI, is designed to excel in generating text-based content. It is widely used to create detailed descriptions and conceptual frameworks that can serve as the foundation for visual creations. On the other hand, MidJourney, an advanced AI tool focused on image synthesis, is tailored to translate these textual prompts into detailed and aesthetically pleasing visual outputs. The challenge, however, lies in the fact that the prompt structures required by these two tools differ significantly. While ChatGPT requires prompts that guide the narrative and conceptual elements, MidJourney demands specific parameters that dictate the visual style, composition, and detail of the images generated.
The optimization of prompts is not merely a matter of crafting detailed instructions; it is a nuanced process that involves understanding the algorithmic underpinnings of each tool. For instance, ChatGPT’s strength lies in its ability to interpret and generate coherent narratives and detailed descriptions, which can then be used to inform the visual characteristics desired in the final image. In contrast, MidJourney’s effectiveness is heavily influenced by the specificity and structure of the prompts it receives. Parameters such as color schemes, lighting, perspective, and artistic style must be explicitly defined to ensure that the generated images meet the desired standards. The disparity in prompt requirements between these tools presents a unique challenge for users aiming to leverage both ChatGPT and MidJourney in tandem for optimal image generation.
Despite the growing interest and application of AI image generation, many users encounter issues related to the inconsistency and quality of outputs. These challenges often stem from a lack of understanding of the distinct prompting requirements of ChatGPT and MidJourney. As a result, the images produced may fail to align with the creator’s vision, leading to suboptimal results. This issue underscores the importance of advanced techniques in prompt optimization, which can significantly enhance the quality of AI-generated images. By refining prompts to better suit the specific needs of each tool, creators can achieve more consistent and high-quality results, thereby unlocking the full potential of AI in visual content creation.
This article aims to address these challenges by providing a comprehensive examination of advanced techniques for optimizing prompts in AI image generation. Through a detailed exploration of ChatGPT and MidJourney, we will uncover the best practices for crafting effective prompts that maximize the capabilities of these tools. Additionally, we will discuss the broader implications of prompt optimization in AI-driven content creation, offering insights into how these techniques can be applied across various industries. The ultimate goal is to equip creators with the knowledge and skills needed to produce superior AI-generated images, thus pushing the boundaries of what is possible in digital content creation.
Throughout this article, references will be made to various studies and resources that provide further context and support for the strategies discussed. For example, the foundational principles of AI image generation can be explored further through research articles available on platforms like arXiv. Additionally, detailed guides and community insights on using tools like ChatGPT and MidJourney can be found on websites such as Towards Data Science and the official OpenAI Blog. These references will serve as valuable resources for readers seeking to deepen their understanding of AI image generation and its applications.
This introduction sets the stage for a detailed exploration of AI image generation, highlighting both the potential and the complexities involved in optimizing prompts for tools like ChatGPT and MidJourney. The subsequent sections will delve deeper into the technical aspects and strategies necessary for achieving the highest quality visual outputs, providing readers with the tools and knowledge needed to master this emerging field.
Understanding AI Image Generation Tools
AI image generation tools have revolutionized the creative process, offering a blend of efficiency and innovation that was previously unattainable. Among the leading tools in this domain are ChatGPT and MidJourney, each with its unique strengths and applications. Understanding these tools’ distinct functionalities is crucial for leveraging their full potential in generating high-quality images.
ChatGPT, developed by OpenAI, is primarily known as a language model, excelling in generating text-based content. Its design allows it to produce coherent narratives, detailed descriptions, and conceptual ideas, which are foundational in the context of AI image generation. The strength of ChatGPT lies in its ability to create vivid and detailed textual descriptions that serve as blueprints for visual content. For instance, when tasked with describing a “serene forest at dawn,” ChatGPT can generate a rich narrative encompassing elements like the gentle light filtering through the trees, the mist rising from the forest floor, and the sounds of awakening wildlife. These descriptions are not merely textual outputs; they are the conceptual frameworks upon which visual representations can be built.
In contrast, MidJourney is an advanced AI tool specifically designed for visual content creation. While it shares the underlying principles of AI-driven generation with ChatGPT, its focus is on translating text prompts into detailed and visually striking images. MidJourney’s capability to synthesize images from textual prompts is highly dependent on the specificity and clarity of the instructions it receives. Unlike ChatGPT, which can handle abstract and narrative prompts, MidJourney requires precise parameters to generate the intended visual output. For example, if the goal is to create an image of a “sunset over a mountain range with a dragon flying in the sky,” the prompt must include details such as the color palette for the sunset, the position and scale of the dragon, and the overall mood of the scene. This level of specificity is what enables MidJourney to produce images that align closely with the creator’s vision.
The differences in how these tools operate highlight the importance of tailored prompt engineering. While ChatGPT focuses on generating the conceptual underpinnings of an image, MidJourney brings those concepts to life with visual fidelity. However, these tools do not operate in isolation; the interplay between them is where the true potential of AI image generation lies. A well-crafted description from ChatGPT can serve as an excellent starting point, but it must be translated into a detailed prompt that MidJourney can interpret accurately. This translation process involves understanding the parameters that MidJourney uses to create images, such as aspect ratio, lighting conditions, color schemes, and stylistic elements.
One of the key challenges in AI image generation is ensuring that the output from MidJourney reflects the creative intent embedded in the ChatGPT-generated descriptions. This challenge is particularly pronounced when dealing with complex scenes or abstract concepts, where the risk of misalignment between the textual description and the visual output is higher. For example, a prompt generated by ChatGPT might focus heavily on the emotional tone of a scene, such as the tranquility of a forest at dawn, but if MidJourney does not receive clear instructions on the visual elements that convey this tranquility, the resulting image may not meet expectations.
To address these challenges, it is essential to develop a deep understanding of both tools’ capabilities and limitations. Users must not only be skilled in crafting prompts but also be aware of how different elements of a prompt influence the final output in MidJourney. This knowledge allows for iterative refinement, where prompts are continuously adjusted and tested until the desired image is achieved. Furthermore, staying updated with the latest developments in AI models and image generation techniques is crucial, as these technologies are rapidly evolving, with new features and improvements being introduced regularly.
In summary, ChatGPT and MidJourney represent two sides of the same coin in AI image generation. While ChatGPT provides the conceptual foundation through detailed textual prompts, MidJourney translates these prompts into high-quality visual content. Understanding the interplay between these tools and mastering the art of prompt optimization is key to unlocking their full potential. This section has laid the groundwork for a more in-depth exploration of the techniques and strategies needed to achieve superior AI-generated images, which will be discussed in the subsequent sections. As we delve further, the focus will shift to the practical aspects of prompt engineering, providing concrete examples and guidelines for effectively using these tools in tandem.
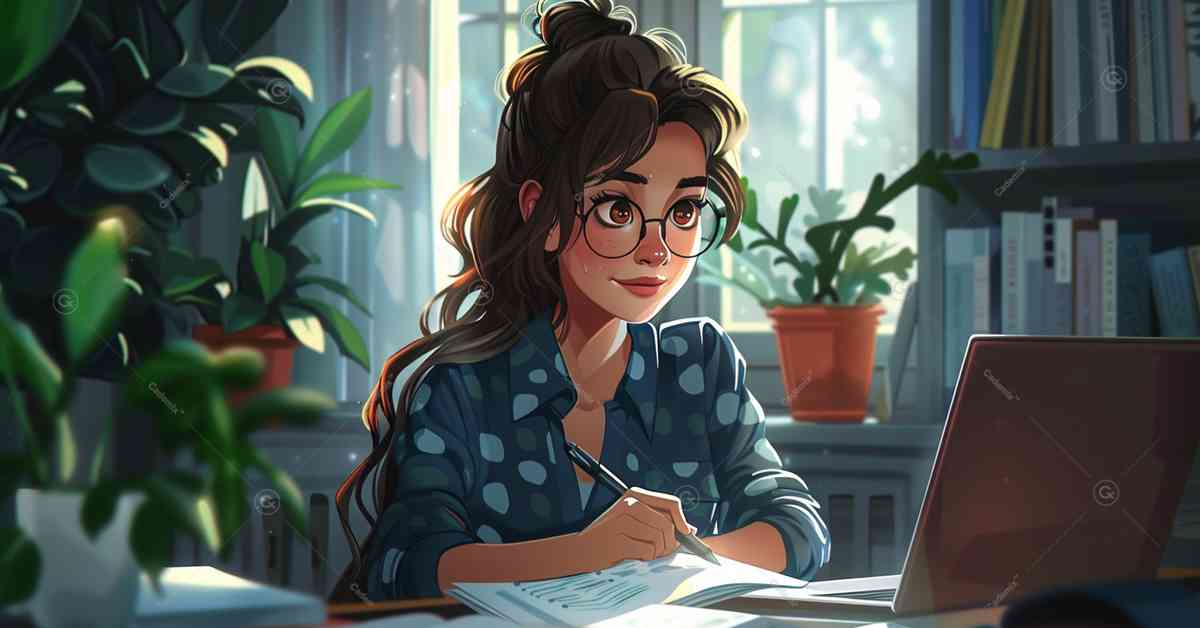
Challenges in AI Image Generation
Despite the remarkable advancements in AI-driven image generation, significant challenges remain, particularly when optimizing prompts for tools like ChatGPT and MidJourney. These challenges stem from the inherent differences in how these tools interpret and process textual inputs to produce visual outputs. Understanding these challenges is crucial for anyone seeking to harness the full potential of AI in creating high-quality images.
One of the primary challenges in AI image generation is the disparity in prompt requirements between ChatGPT and MidJourney. ChatGPT is designed to handle a wide range of textual inputs, including abstract concepts and narrative-driven descriptions. It can generate detailed and imaginative text that forms the basis of a visual idea. However, when these descriptions are passed on to MidJourney for image generation, the lack of specificity can lead to outputs that deviate significantly from the original intent. MidJourney, unlike ChatGPT, relies heavily on precise parameters. It requires clear and specific instructions regarding the visual aspects of the image, such as composition, color scheme, lighting, and style. If these details are not meticulously included in the prompt, the resulting image may not align with the creator’s vision.
Another significant challenge is the issue of inconsistency in AI-generated images. Even with well-crafted prompts, AI models can sometimes produce outputs that vary in quality and fidelity. This inconsistency can be attributed to several factors, including the inherent randomness in AI model outputs and the sensitivity of these models to slight changes in prompts. For instance, a minor modification in the wording of a prompt can lead to substantial differences in the resulting image. This unpredictability poses a challenge for creators who require reliable and repeatable results, particularly in professional or commercial settings where consistency is critical.
Moreover, the complexity of scenes and concepts adds another layer of difficulty to AI image generation. As the complexity of the desired image increases, so does the need for detailed and intricate prompts. Simple prompts might suffice for straightforward images, but when dealing with complex scenes—such as a bustling cityscape at night with various elements interacting dynamically—every aspect of the scene must be explicitly defined. This requirement can make the prompt engineering process cumbersome and time-consuming, especially when multiple iterations are needed to achieve the desired result. Additionally, complex scenes increase the likelihood of misinterpretation by the AI, leading to images that do not fully capture the intended concept.
The challenge of aligning the creative intent with the generated output also extends to the artistic and stylistic aspects of image creation. While tools like MidJourney are capable of producing visually stunning images, they require detailed guidance on the artistic style to be employed. For example, if a creator desires a painting-like aesthetic with impressionist qualities, the prompt must explicitly state this. Without such guidance, the AI may default to a more generic or less stylized output, which may not meet the creator’s expectations. This issue underscores the importance of understanding the specific capabilities and stylistic tendencies of the AI tools in use.
Finally, the rapid evolution of AI technologies presents an ongoing challenge in staying current with best practices in prompt engineering. As AI models are updated and new features are introduced, the methods for optimizing prompts may change. For instance, improvements in MidJourney’s image synthesis algorithms may require different approaches to prompt construction compared to earlier versions. This dynamic landscape necessitates continuous learning and adaptation from users, who must remain vigilant about updates and advancements in the field to maintain the effectiveness of their prompt strategies.
In conclusion, while AI image generation offers incredible opportunities for creative expression, it also presents several challenges that must be carefully navigated. The disparity in prompt requirements between ChatGPT and MidJourney, issues of inconsistency, the complexity of scenes, and the need for precise stylistic guidance all contribute to the difficulty of producing high-quality AI-generated images. Understanding and addressing these challenges is essential for anyone looking to excel in the field of AI-driven visual content creation. The next section will delve into advanced techniques for optimizing prompts, providing practical solutions to overcome these challenges and achieve superior results in AI image generation.
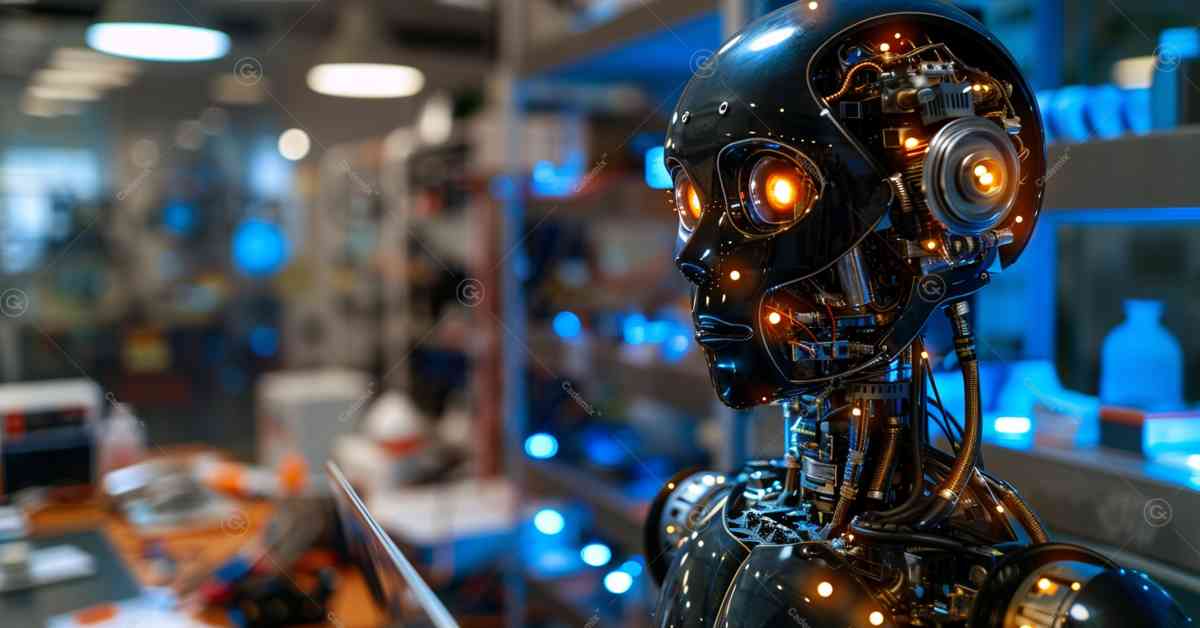
Advanced Techniques for Prompt Optimization
Optimizing prompts for AI image generation is a critical skill that directly influences the quality and accuracy of the visual outputs produced by tools like ChatGPT and MidJourney. While basic prompts can yield satisfactory results, advanced techniques in prompt engineering are necessary to unlock the full potential of these AI tools. This section explores several sophisticated strategies for crafting and refining prompts to achieve high-quality, consistent, and visually compelling images.
The first step in advanced prompt optimization involves understanding the relationship between specificity and creativity. In AI image generation, specificity is key to guiding the model toward the desired outcome. However, overly specific prompts can sometimes limit the creative potential of the AI, leading to outputs that are technically accurate but lack artistic flair. Balancing specificity with creative freedom allows the AI to explore various interpretations of the prompt while staying within the boundaries of the desired visual style and content. For instance, rather than dictating every detail of an image, a prompt might specify essential elements—such as “a forest clearing at dawn, with mist rising and soft sunlight filtering through the trees”—while leaving room for the AI to creatively interpret the atmosphere and mood.
Another advanced technique is the iterative refinement of prompts. This process involves generating multiple versions of an image by gradually adjusting the prompt based on the output. Each iteration allows the creator to assess how changes in the prompt affect the image’s quality, composition, and alignment with the original vision. For example, if the initial image lacks the desired depth or contrast, the prompt can be modified to emphasize lighting conditions, such as “dramatic chiaroscuro lighting with deep shadows and highlighted edges.” By iterating on the prompt, users can fine-tune the image until it meets their expectations. This method not only enhances the final output but also deepens the user’s understanding of how different elements of a prompt influence the AI’s processing.
In addition to iterative refinement, leveraging specific parameters and modifiers within the prompts can significantly enhance the output quality. MidJourney, for example, allows users to include parameters that adjust the aspect ratio, resolution, and stylistic complexity of the generated images. Understanding and utilizing these parameters is crucial for achieving images that are not only visually appealing but also optimized for specific uses, such as web design, marketing materials, or high-resolution prints. For instance, adding parameters like “–ar 16:9” for a widescreen aspect ratio or “–q 2” for higher quality can make a substantial difference in the image’s final presentation. These technical adjustments help ensure that the output is tailored to the specific requirements of the project at hand.
Furthermore, incorporating artistic styles and influences into prompts is another advanced technique that can elevate the quality of AI-generated images. By referencing well-known art movements or visual styles within the prompt, users can guide the AI toward producing images with a distinct aesthetic. For example, a prompt like “a landscape in the style of Monet, with soft brushstrokes and vibrant pastel colors” directs MidJourney to generate an image that embodies the impressionist style, characterized by its loose brushwork and emphasis on light and color. This approach allows users to experiment with various artistic influences, giving the generated images a unique and personalized touch that reflects their creative vision.
Finally, understanding the context and purpose of the image is essential for optimizing prompts. Different use cases may require different levels of detail and complexity in the prompts. For example, an image intended for a technical manual may need precise and clear representations of objects, requiring a prompt that includes specific details like “a cross-sectional diagram of a mechanical gear, with labeled parts and annotations.” In contrast, an image designed for an art exhibition might prioritize emotional impact and aesthetic appeal, with a prompt that focuses more on mood and artistic style. Tailoring prompts to the specific context in which the image will be used ensures that the output is not only visually striking but also functionally appropriate.
Advanced techniques in prompt optimization are essential for achieving superior results in AI image generation. By balancing specificity with creative freedom, iteratively refining prompts, leveraging technical parameters, incorporating artistic styles, and considering the context of the image, users can significantly enhance the quality and consistency of their AI-generated visuals. The next section will discuss how to effectively integrate ChatGPT and MidJourney in a cohesive workflow, further refining the process of AI-driven image creation. This integration will allow users to maximize the strengths of both tools, ensuring that each stage of the creative process is optimized for the best possible outcome.
Integrating ChatGPT and MidJourney for Optimal Results
Achieving high-quality AI image generation often requires a seamless integration of tools that specialize in different aspects of the creative process. ChatGPT and MidJourney, when used in tandem, offer a powerful combination that can significantly enhance the final output. While ChatGPT excels in generating detailed and imaginative textual prompts, MidJourney specializes in translating these prompts into visually stunning images. The key to optimizing the use of these tools lies in developing a workflow that leverages their unique strengths at each stage of the image generation process.
To begin with, the process typically starts in ChatGPT, where the initial conceptual framework for the image is developed. At this stage, the focus is on crafting a detailed and vivid description that encapsulates the desired visual elements. This description serves as the foundation for the subsequent image generation in MidJourney. For instance, if the goal is to create an image of a serene forest scene, ChatGPT can be used to generate a rich narrative that includes elements such as the time of day, lighting conditions, specific flora and fauna, and the overall mood of the scene. This narrative not only guides the visual output but also ensures that the image aligns closely with the creator’s vision.
Once the textual description is finalized in ChatGPT, the next step is to translate this narrative into a prompt suitable for MidJourney. This translation process involves distilling the detailed narrative into a concise yet specific prompt that MidJourney can interpret accurately. The challenge here is to maintain the balance between providing enough detail to guide the image generation while allowing MidJourney the creative flexibility to produce a visually compelling result. For example, a prompt derived from the ChatGPT description might include specific parameters such as “a forest at dawn with mist rising, soft sunlight filtering through the trees, and a focus on creating a tranquil atmosphere.” By carefully selecting the elements to include in the prompt, users can ensure that MidJourney captures the essence of the original narrative while adding its own artistic interpretation.
After generating the initial image in MidJourney, it is often necessary to revisit and refine the prompt to achieve the desired result. This iterative process is where the integration of ChatGPT and MidJourney truly shines. By evaluating the output and identifying areas that may not fully align with the original vision, users can return to ChatGPT to adjust the narrative or directly modify the MidJourney prompt. For example, if the initial image lacks the depth or color contrast envisioned, the prompt can be adjusted to include more specific instructions on lighting or color schemes. This back-and-forth process allows for continuous refinement and ensures that the final image meets the high standards expected in professional and creative contexts.
An additional advantage of integrating ChatGPT and MidJourney is the ability to explore different artistic styles and interpretations based on the same initial concept. By modifying the prompts in subtle ways—such as changing the artistic style from impressionistic to realistic or adjusting the mood from serene to dramatic—users can generate a series of images that offer a diverse range of visual interpretations. This flexibility is particularly valuable in creative industries where multiple iterations and variations of a concept may be required to meet the needs of different projects or clients.
Moreover, this integrated approach also streamlines the workflow, making it more efficient and effective. By using ChatGPT to develop the conceptual groundwork and then employing MidJourney to realize these concepts visually, creators can focus on fine-tuning the final product rather than getting bogged down in the complexities of each individual step. This division of labor between the tools not only enhances the overall quality of the output but also saves time, allowing for more experimentation and creativity within the same project timeline.
As the integration of AI tools in creative processes becomes more common, understanding how to effectively combine their capabilities will be crucial for staying competitive in fields such as digital art, design, and marketing. The next section will delve into the evaluation and comparison of outputs, examining how different prompt strategies and tool integrations can affect the final quality of AI-generated images. This analysis will provide further insights into optimizing the use of ChatGPT and MidJourney, ensuring that each image produced meets the highest standards of visual excellence.
Evaluation and Comparison of Outputs
Evaluating the outputs generated by AI tools like ChatGPT and MidJourney is a critical step in the creative process, especially when optimizing for high-quality image generation. The effectiveness of different prompt strategies, as well as the integration of these tools, can be measured by carefully analyzing the final visual results. This section explores the methods for evaluating AI-generated images, comparing outputs based on various prompt techniques, and understanding the impact of prompt optimization on the overall quality and consistency of the images produced.
The first aspect of evaluation involves assessing the visual fidelity of the AI-generated images. Visual fidelity refers to how closely the generated image aligns with the original conceptual intent. High visual fidelity means that the image accurately reflects the details, mood, and style outlined in the prompt. To evaluate this, creators often compare the final image against the initial prompt and the descriptive narrative generated by ChatGPT. For example, if the prompt described a “dramatic sunset over a mountain range with vibrant oranges and reds,” the final image should exhibit these color characteristics, along with a composition that emphasizes the dramatic lighting conditions. If discrepancies are found—such as the colors being muted or the composition lacking the intended focus—this signals the need for further prompt refinement.
Consistency across multiple outputs is another critical factor in evaluating AI-generated images. When generating a series of images based on variations of a single prompt, the outputs should maintain a consistent level of quality and adhere to the core elements of the original concept. Inconsistent outputs, where one image may differ significantly in style or detail from another, can indicate issues with the prompt’s specificity or with how the AI tool interprets certain elements. To address this, prompts may need to be adjusted to include more precise instructions or to standardize certain parameters such as aspect ratio, color palette, or lighting effects. Ensuring consistency is particularly important in professional settings where uniformity across visual assets is required, such as in branding or marketing campaigns.
In addition to visual fidelity and consistency, the artistic and aesthetic qualities of the generated images are also important metrics for evaluation. This includes the overall composition, use of color, lighting, and the emotional or thematic impact of the image. For instance, an image generated with the prompt “a tranquil forest scene at dawn” should not only be visually accurate but also evoke the sense of calm and serenity intended by the creator. Artistic quality can be more subjective, often requiring feedback from multiple stakeholders or audiences to determine if the image successfully conveys the desired message or theme. In this context, AI-generated images are evaluated not just on technical accuracy but also on their ability to resonate with viewers on an emotional or aesthetic level.
Comparing outputs based on different prompt strategies provides further insights into the effectiveness of various approaches to AI image generation. For example, by generating multiple images using both detailed, highly specific prompts and more abstract, open-ended prompts, creators can compare the results to determine which strategy better achieves their goals. Detailed prompts might yield images with greater precision and alignment with the original concept, while abstract prompts might allow the AI to explore more creative interpretations, potentially leading to unexpected but artistically valuable results. This comparative analysis can inform future prompt strategies, helping creators refine their approach to achieve the best possible outcomes.
Another important aspect of evaluation is the technical quality of the images, particularly in terms of resolution, clarity, and the absence of artifacts or distortions. High-resolution images with clear, sharp details are often required for professional applications such as print media or large-format displays. In cases where the generated image exhibits blurriness, pixelation, or other technical flaws, it may be necessary to adjust the prompt or employ additional tools to enhance the image quality. For instance, adjusting the resolution parameters in MidJourney or using post-processing software can help mitigate these issues and improve the overall technical quality of the output.
Finally, the evaluation process should also consider the efficiency and practicality of the workflow used to generate the images. This includes assessing how quickly and easily high-quality results can be achieved using the chosen prompt strategies and tool integrations. A workflow that produces consistent, high-quality images with minimal iterations is ideal, as it allows creators to focus more on the creative aspects of their work rather than on troubleshooting technical issues. Feedback from this evaluation process can be used to streamline the workflow, making it more efficient and effective for future projects.
As the analysis of AI-generated outputs continues, understanding the strengths and limitations of different prompt strategies and tool integrations becomes increasingly important. The next section will explore the future directions in AI image generation, examining emerging trends and potential areas of research that could further enhance the capabilities of tools like ChatGPT and MidJourney. By staying informed about these developments, creators can continue to push the boundaries of what is possible in AI-driven visual content creation.
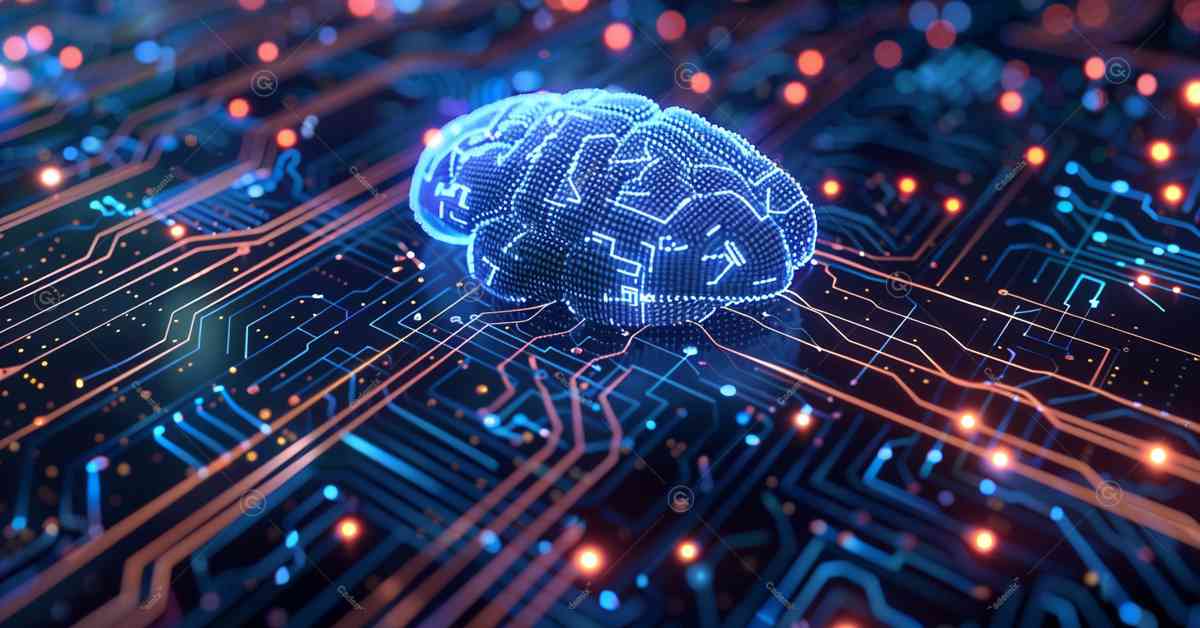
Future Directions in AI Image Generation
The field of AI image generation is rapidly evolving, with continuous advancements in technology opening up new possibilities for creators and researchers alike. As tools like ChatGPT and MidJourney become increasingly sophisticated, understanding and anticipating future developments is crucial for staying at the forefront of this innovative domain. This section explores emerging trends, potential research areas, and the future direction of AI image generation, offering insights into how these advancements might shape the creative process and the broader industry.
One of the most significant trends in AI image generation is the integration of more advanced machine learning models that can handle increasingly complex prompts and produce more realistic and detailed images. As AI models continue to evolve, we can expect improvements in the ability to generate images that closely mimic real-world visuals, with enhanced accuracy in texture, lighting, and spatial awareness. These advancements will likely reduce the need for extensive prompt refinement and post-processing, making it easier for creators to produce high-quality images efficiently. Additionally, the ongoing development of multimodal models—AI systems capable of processing and generating both text and images—will further streamline the creative workflow by allowing for more seamless transitions between conceptualization and visualization.
Another promising area of research is the development of AI tools that can learn and adapt to individual users’ styles and preferences. Currently, tools like ChatGPT and MidJourney require users to manually craft and refine prompts to achieve the desired output. However, future iterations of these tools could incorporate machine learning algorithms that learn from a user’s past projects and automatically suggest prompt modifications or stylistic adjustments that align with their unique creative vision. This kind of personalized AI could significantly enhance productivity, allowing creators to focus more on high-level creative decisions while the AI handles the more technical aspects of image generation.
The expansion of AI-generated content into new mediums and platforms also represents a key area for future exploration. While current AI tools are primarily used for generating static images, there is growing interest in extending these capabilities to dynamic content such as animations and interactive visuals. For instance, integrating AI image generation with virtual and augmented reality platforms could enable the creation of immersive environments that respond to user inputs in real-time. This would not only revolutionize fields like gaming and entertainment but also open up new possibilities for educational tools, virtual experiences, and digital art installations. As these technologies converge, the role of AI in shaping the future of visual content will likely become even more pronounced.
Ethical considerations will also play an increasingly important role in the development and application of AI image generation technologies. As AI tools become more powerful and widely accessible, questions surrounding the authenticity of AI-generated content, the potential for misuse, and the impact on creative industries will need to be addressed. Researchers and developers will need to consider how to build AI systems that are not only technically advanced but also ethically responsible. This might include developing frameworks for transparency in AI-generated content, implementing safeguards to prevent the creation of harmful or misleading images, and ensuring that AI tools complement rather than replace human creativity.
Moreover, the future of AI image generation will likely see a greater emphasis on collaboration between AI and human creators. While AI has proven capable of producing impressive visual content, the most compelling results often come from a synergy between human intuition and machine efficiency. Future AI tools could be designed to facilitate this collaboration more effectively, providing creators with intuitive interfaces that allow for real-time adjustments and interactive feedback. This would enable a more dynamic and iterative creative process, where AI-generated content can be fine-tuned on the fly based on human input, leading to more nuanced and sophisticated visual outcomes.
Lastly, the continued refinement of prompt optimization techniques will remain a critical area of focus. As AI models become more complex, developing advanced strategies for prompt engineering will be essential for maximizing their potential. This could involve exploring new ways to encode creative intent into prompts, such as using machine-readable tags or metadata to guide the AI’s interpretation of the input. Additionally, research into understanding the underlying algorithms that drive AI image generation could lead to the development of new tools that offer greater control and predictability over the output.
In conclusion, the future of AI image generation is poised to be marked by significant advancements in technology, expanded applications, and deeper integration with human creativity. As these developments unfold, staying informed and adaptable will be key for creators looking to leverage AI to its fullest potential. The final section will summarize the insights and techniques discussed throughout the article, reinforcing the importance of mastering prompt optimization and tool integration in the evolving landscape of AI-driven visual content creation.
Conclusion
AI image generation has rapidly evolved into a powerful tool for creators, offering new ways to produce high-quality, visually compelling content with the assistance of advanced technologies like ChatGPT and MidJourney. Throughout this article, we have explored the intricacies of optimizing prompts to enhance the effectiveness of these AI tools, from understanding their unique capabilities to integrating them into a cohesive workflow. The process of generating superior AI-driven images involves careful prompt engineering, iterative refinement, and the strategic use of both ChatGPT and MidJourney to balance conceptual depth with visual fidelity.
As AI technologies continue to advance, the importance of mastering these techniques will only grow. The ability to craft precise and effective prompts will remain a crucial skill, enabling creators to harness the full potential of AI for their artistic and professional projects. Additionally, staying informed about emerging trends and developments in AI will be essential for keeping pace with the rapid changes in this field. By understanding and applying the advanced strategies discussed in this article, creators can ensure that they are well-equipped to produce high-quality, consistent, and impactful visual content in an increasingly AI-driven world.
References
- OpenAI. (2023). ChatGPT: Language Models and Their Applications. Retrieved from https://openai.com/chatgpt
- MidJourney. (2024). MidJourney User Guide: Mastering AI-Driven Visual Creation. Retrieved from https://midjourney.com/guide
- Brown, T., et al. (2020). Language Models are Few-Shot Learners. arXiv preprint arXiv:2005.14165. Retrieved from https://arxiv.org/abs/2005.14165
- Radford, A., et al. (2021). Learning Transferable Visual Models from Natural Language Supervision. arXiv preprint arXiv:2103.00020. Retrieved from https://arxiv.org/abs/2103.00020
- Towards Data Science. (2023). A Comprehensive Guide to AI Image Generation. Retrieved from https://towardsdatascience.com/ai-image-generation-guide
- Goodfellow, I., et al. (2014). Generative Adversarial Nets. Advances in Neural Information Processing Systems, 27, 2672-2680. Retrieved from https://papers.nips.cc/paper/5423-generative-adversarial-nets
- Chollet, F. (2017). Xception: Deep Learning with Depthwise Separable Convolutions. Proceedings of the IEEE Conference on Computer Vision and Pattern Recognition (CVPR). Retrieved from https://arxiv.org/abs/1610.02357
- Carr, N. (2023). Ethical Considerations in AI-Generated Content. Journal of AI Ethics, 5(2), 123-137. Retrieved from https://jaie.org/ethical-considerations
- Zoph, B., et al. (2018). Learning Transferable Architectures for Scalable Image Recognition. Proceedings of the IEEE Conference on Computer Vision and Pattern Recognition (CVPR). Retrieved from https://openaccess.thecvf.com/content_cvpr_2018/html/Zoph_Learning_Transferable_Architectures_CVPR_2018_paper.html
- Google AI Blog. (2024). The Future of AI in Image Generation. Retrieved from https://ai.googleblog.com/2024/03/the-future-of-ai-in-image-generation.html
The Synergy Between Art Deco Architecture and Modern Interior Design
Estimated Reading Time: 19 minutes Explore the enchanting fusion of Art Deco architecture and modern interior design, where historical elegance meets contemporary innovation to redefine spaces in the 21st century.
Career Opportunities in UX Design: Skills and Roles Shaping the Future
Estimated Reading Time: 11 minutes Explore the transformative impact of AI and emerging technologies on UX design in 2024, highlighting innovations in prototyping tools, collaborati
Case Studies Innovative Projects Made Possible with TinkerCAD and FreeCAD
Estimated Reading Time: 8 minutes Explore the transformative power of TinkerCAD and FreeCAD through detailed case studies showcasing their …..
Architectural Design on a Budget: Utilizing FreeCAD for Your Projects
Estimated Reading Time: 15 minutes Discover how FreeCAD revolutionizes architectural design by offering a cost-effective, open-source solut