Abstract
The reliability and efficiency of high-voltage power systems are crucial for stable electricity supply in modern energy infrastructures. With the increasing integration of renewable energy sources and the rising demand for electrical energy, grid operators face the challenge of minimizing failures and optimizing maintenance costs. AI-driven predictive maintenance offers innovative solutions to predict and prevent failures by using advanced algorithms and data analytics. This article explores the role of AI in predictive maintenance of high-voltage power systems, highlighting the benefits, challenges, and future developments of this technology.
By: Alireza Alidadi, Cademix Institute of Technology Austria
Table of Contents
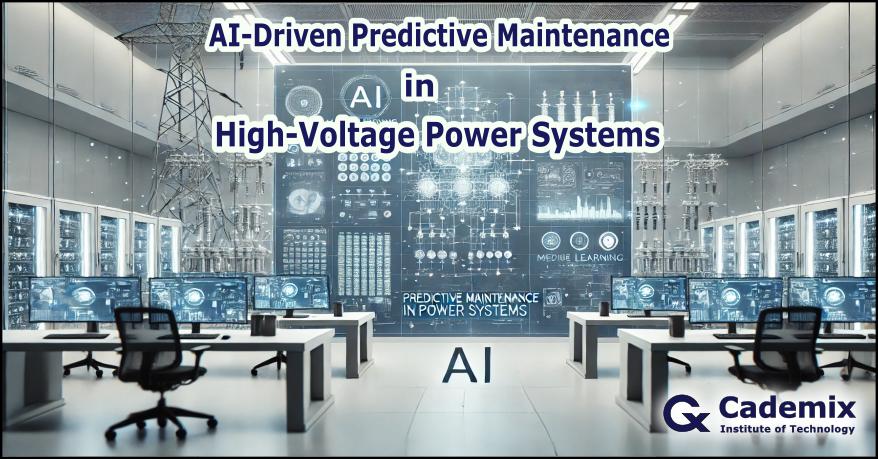
1. Introduction
High-voltage power systems are the backbone of modern power grids, transmitting large amounts of electricity over long distances to meet the needs of households, businesses, and industries. The reliable operation of these systems is critical, as failures can have significant economic and social impacts. Traditional maintenance methods, based on periodic inspections and reactive repairs, are increasingly reaching their limits. Given the complexity and size of modern power grids, there is a growing need for more efficient and precise maintenance strategies.
Predictive maintenance has emerged as a promising approach to enhance the reliability of high-voltage power systems. Unlike reactive or preventive maintenance approaches, which respond to existing problems or rely on regular maintenance intervals, predictive maintenance aims to detect and address potential issues before they lead to failures. This is achieved through the analysis of real-time data from various sensors and monitoring systems integrated into the power grid.
Artificial Intelligence (AI) plays a key role in predictive maintenance by using advanced algorithms for pattern recognition, anomaly detection, and prediction. By processing large amounts of data and applying machine learning techniques, AI systems can make accurate predictions about the condition of high-voltage power systems and provide recommendations for maintenance actions. This article explores the various aspects of AI-driven predictive maintenance, including its benefits, challenges, and application possibilities.
2. Importance of Predictive Maintenance in High-Voltage Power Systems
Predictive maintenance is essential for maintaining the reliability and efficiency of high-voltage power systems. These systems are exposed to constant stress, including electrical load fluctuations, weather conditions, and wear and tear. A failure of a high-voltage component, such as a transformer, line, or switch, can have far-reaching consequences, from power outages to significant economic losses. Predictive maintenance helps to minimize such risks by enabling early detection of problems.
By implementing predictive maintenance, grid operators can significantly reduce downtime. Instead of waiting for a component to fail and then carrying out repairs, predictive maintenance allows for proactive maintenance planning. Sensors and monitoring systems continuously collect data on the condition of components, and AI algorithms analyze this data to identify signs of wear or impending failures. This early detection enables maintenance teams to intervene in a timely manner and fix issues before they escalate into major problems.
Another critical aspect of predictive maintenance is cost optimization. Traditional maintenance approaches, which rely on fixed inspection intervals, often lead to unnecessary maintenance and associated costs. By using predictive maintenance, maintenance activities can be carried out precisely when they are needed. This not only reduces maintenance costs but also extends the lifespan of components by avoiding unnecessary interventions. The savings from predictive maintenance are particularly significant in large-scale high-voltage power systems, where the costs of downtime and repairs can be high.
3. Challenges of Predictive Maintenance
Despite the significant benefits of predictive maintenance, there are also challenges that must be addressed during implementation. One of the biggest challenges is data integration. High-voltage power systems consist of various components that generate different types of data, such as temperature, voltage, current, and mechanical stress. Integrating and analyzing these heterogeneous data sources requires advanced data management systems and technologies to deliver accurate and actionable insights.
Another issue is the accuracy and reliability of predictions. AI models used for predictive maintenance must be trained on extensive and high-quality datasets to make accurate predictions. The quality of the data is crucial, as inaccurate or incomplete data can lead to incorrect predictions, which in turn can result in unnecessary maintenance actions or even unexpected failures. Therefore, it is important to continuously monitor and improve data quality.
Additionally, security and privacy concerns pose a challenge. Collecting and processing large amounts of operational data involves risks related to the security and protection of sensitive information. Grid operators must ensure that their predictive maintenance systems are robust against cyber-attacks and that data processing complies with privacy regulations. This requires the use of advanced security technologies and protocols to ensure the integrity and confidentiality of the data.
4. AI Technologies in Predictive Maintenance
Artificial Intelligence offers a range of technologies and methods that can be used for predictive maintenance of high-voltage power systems. Key AI technologies include machine learning, deep learning, and natural language processing (NLP). These technologies enable the analysis of large datasets, recognition of patterns, and prediction of the condition of components and systems.
4.1 Machine Learning
Machine learning is one of the most commonly used AI technologies in predictive maintenance. It involves algorithms that learn from historical data and make predictions based on that information. In high-voltage power systems, machine learning algorithms are used to detect anomalies in operational data that indicate potential faults or failures. Through continuous learning and adaptation, these algorithms can improve their accuracy over time.
A common approach in machine learning is the use of supervised learning, where algorithms are trained on labeled datasets. These datasets contain examples of normal and abnormal operating conditions, enabling the algorithm to distinguish between them. Another important concept is unsupervised learning, where algorithms identify patterns in unlabeled data to detect unknown anomalies or new types of faults. Both approaches can provide valuable insights into the condition of high-voltage power systems and indicate potential issues early on.
4.2 Deep Learning
Deep learning is a specialized form of machine learning that uses deep neural networks to recognize complex patterns in large datasets. This technology is particularly useful for processing and analyzing data from various sources, such as sensor data, images, and time series. In high-voltage power systems, deep learning can be used to create detailed models of system states and make accurate predictions about the remaining lifespan of components.
A significant advantage of deep learning is its ability to learn from unstructured data. This is especially important in high-voltage power systems, where data often comes in different formats and structures. By using deep neural networks, systems can identify complex relationships and dependencies between data that are difficult to detect with conventional methods. This leads to more accurate predictions and better decision support for maintenance actions.
However, implementing deep learning requires substantial computing resources and specialized knowledge. The models must be carefully designed and trained to avoid overfitting and ensure high accuracy. This means that grid operators must invest in powerful hardware and software, as well as in training specialized personnel, to fully exploit the benefits of deep learning.
4.3 Natural Language Processing (NLP)
Natural Language Processing (NLP) is another AI technology used in predictive maintenance of high-voltage power systems. NLP enables computers to understand and process human language, which is useful for analyzing text data, such as maintenance logs, inspection reports, and fault descriptions. By analyzing these texts, AI systems can gain additional information about the condition of components and potential issues.
NLP technologies can also be used to automatically generate reports and prioritize maintenance tasks. By combining NLP with machine learning, systems can extract the most relevant information from large amounts of text data and help maintenance teams focus on the most urgent tasks. This improves the efficiency and responsiveness of maintenance and helps prevent failures.
Furthermore, NLP can be used to enhance communication between humans and machines. Voice-activated interfaces allow maintenance personnel to quickly and easily access information without having to use complex computer applications. This facilitates access to critical data and contributes to the efficiency and safety of maintenance operations.
5. Application Examples
5.1 Use of AI in the European Energy Sector
In Europe, many energy providers are already using AI-driven predictive maintenance systems to improve the reliability and efficiency of their high-voltage power grids. For example, a large European transmission system operator uses machine learning to analyze data from transformers, lines, and switches. By monitoring parameters such as temperature, humidity, and current load, the system can detect anomalies and provide early warnings of potential problems.
Another significant use of AI can be observed in offshore wind farms. These facilities are often difficult to access and expensive to maintain, making predictive maintenance particularly valuable. By using sensors and drones to collect real-time data, and AI algorithms to analyze this data, operators can optimize maintenance activities and maximize the availability of wind turbines. The results show that the introduction of AI-driven predictive maintenance systems has led to significant cost savings and improved reliability of power supply.
5.2 AI-Based Maintenance in the United States
In the United States, several major energy providers are leveraging AI-based predictive maintenance to improve the reliability and efficiency of their high-voltage grids. One example is the use of AI to monitor and analyze transmission lines. By using drones and satellites to capture image data, and then analyzing these images using machine learning, utilities can identify signs of wear, weather-related damage, or vegetation encroachment that could potentially lead to failures.
Additionally, some companies use AI to monitor transformers and substations. These components are crucial for grid stability and can have significant impacts if they fail. AI-driven predictive maintenance allows operators to continuously monitor the condition of these devices and identify anomalies before they lead to major issues. This proactive maintenance strategy helps utilities minimize downtime and extend the lifespan of assets.
5.3 Predictive Maintenance in Asia
In Asia, particularly in countries like China and Japan, AI-driven predictive maintenance is increasingly used in high-voltage power systems. In China, for example, the state grid operator has developed a comprehensive strategy to implement AI-driven predictive maintenance systems to improve the efficiency and reliability of the vast power grid. This includes monitoring transmission lines using drones and analyzing sensor data from substations and transformers.
Japanese energy companies are also adopting predictive maintenance to address the challenges of aging energy infrastructure. AI systems continuously analyze operational data from high-voltage equipment and identify potential risks before they lead to failures. The integration of AI into maintenance strategies has helped reduce operational costs and enhance the reliability of power supply, which is particularly important in a country with high energy supply demands like Japan.
6. Conclusion
AI-driven predictive maintenance offers significant benefits for managing and optimizing high-voltage power systems. By detecting and predicting issues early, grid operators can reduce downtime, lower maintenance costs, and improve the reliability of power supply. The application of AI technologies, such as machine learning, deep learning, and NLP, enables precise analysis of large datasets and provides valuable insights into the condition of infrastructure.
Despite challenges such as data integration, prediction accuracy, and security concerns, the practice shows that implementing AI-driven predictive maintenance systems is feasible and beneficial. The ongoing development of these technologies will further enhance the efficiency and reliability of high-voltage power systems and help meet the growing demands for stable and sustainable energy supply.
The future of predictive maintenance in high-voltage power systems will be shaped by continuous innovations in AI technology. With advancements in sensors, data analytics, and computing power, the ability for predictive maintenance will be further refined and expanded. This will enable grid operators to manage their infrastructures more efficiently and successfully address the challenges of modern energy supply.
7. Future Outlook
The future of AI-driven predictive maintenance in high-voltage power systems looks promising as the technology continues to evolve. Future advancements in sensors, data processing, and AI algorithms will further improve the capability of predictive maintenance. For example, advances in the Internet of Things (IoT) and 5G technology could enable even more comprehensive and detailed data collection, leading to more accurate predictions and better maintenance strategies.
Moreover, advancements in AI research will open up new possibilities to make predictive maintenance systems even more effective. The development of advanced algorithms for machine learning and deep learning will allow for better detection of complex patterns and anomalies in data, resulting in more precise predictions. These technologies will help grid operators better understand their systems and make more efficient maintenance decisions.
However, the integration of AI-driven predictive maintenance into the daily operation of high-voltage power systems requires close collaboration between technology providers, grid operators, and regulatory authorities. Common standards and protocols need to be developed to ensure the interoperability and security of the systems. Only through a coordinated effort of all stakeholders can the full potential of AI-driven predictive maintenance be realized to improve the reliability and efficiency of modern power supply.
References
- McKinsey & Company. (2021). Harnessing AI for Predictive Maintenance in Power Systems.
- International Energy Agency (IEA). (2020). Digitalization and Energy.
- Siemens AG. (2022). AI in Energy: Transforming the Future of Power Systems.
- IEEE Power & Energy Society. (2019). AI-Based Predictive Maintenance in High-Voltage Transmission.
- State Grid Corporation of China. (2021). Applying AI for Smart Grid Operations and Maintenance.
- European Commission. (2020). Artificial Intelligence in the Energy Sector: Opportunities and Challenges.